Main Second Level Navigation
Breadcrumbs
- Home
- News & Events
- Recent News
- Dear Silo Buster: We Hear a Lot About Artificial Intelligence ....
Dear Silo Buster: We Hear a Lot About Artificial Intelligence ....
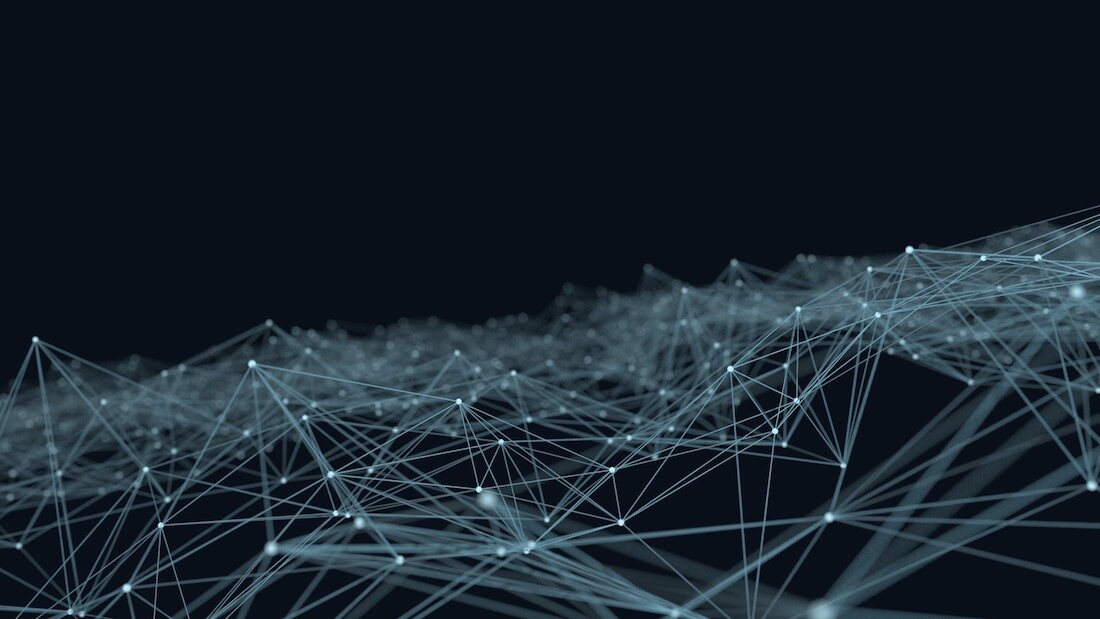
Silo Buster is a new occasional feature highlighting people, places and projects crossing U of T Med domains and disciplines to advance collaboration, research and impact. Send your story ideas to medicine.communications@utoronto.ca.
Dear Silo Buster: We hear a lot about artificial intelligence as “the next big thing” in healthcare. What’s going on at U of T’s affiliated hospitals in applying AI directly to patient care?
It’s true, there’s a whole lot of hype going on around AI and healthcare, particularly in the U.S.
Here at home, there are some promising machine-learning projects percolating at university-affiliated hospitals in the GTA. To get a handle on who’s doing what, Muhammad Mamdani at St. Michael’s Hospital is organizing Toronto’s first summit – Data Analytics for Sustaining Hospitals on Dec. 3 – to share on-the-ground information between data leaders such as CIOs and decision support teams across hospitals.
One of the projects Mamdani’s team is working on is training a deep-learning algorithm to constantly monitor patients to predict in advance which high-risk inpatients could take a turn for the worse. This algorithm has trained on millions of routinely collected data points from over 20,000 patients and is yielding very high levels of accuracy. The goal is to get this information into the hands (and mobile devices) of physicians before bad things happen.
St. Michael’s will be the first hospital in Canada to launch this type of “early warning system” in their internal medicine units. How did Mamdani get this silo-busting project going? Here’s the back story and his tips for success.
--
For Mamdani it began with a conversation in 2015 with clinical leaders and hospital management in which a shared understanding emerged: making data-informed clinical decisions is incredibly difficult as it is a real struggle to get the right data into the right hands at the right time.
Clinicians and staff certainly collect a lot of data about patients – lab values, medication doses, imaging results etc. They may “check a lot of boxes” but these data are not collected to be used for advanced analytical purposes. That was a key insight for Mamdani.
“It was really one conversation where the lightbulb went off. The data we have is too cumbersome. The quality may be questionable. It takes too long to get. And we often can’t quickly understand what we are receiving.”
This is a serious problem for busy hospitals with heavy and complex caseloads. Data needs to work for clinical decisionmakers and their patients, not against them. Here’s how Mamdani and his team of data scientists at the Li Ka Shing Centre for Healthcare Analytics Research & Training (LKS-CHART) are tackling this:
Start with a big step back and envision the future
Mamdani and his colleagues – computer scientists, engineers, statisticians, clinicians, and administrators – want to see a system where clinicians don’t have to think twice when they’re going about their day: self-running dashboards with rigorously tested algorithms sifting, weighing and making predictions based on real-time patient data flowing into the system. Those systems should then push relevant pop-up alerts to specific clinicians, targeting them with valuable information: data they need when they need it; analytics they are confident to act on.
Build a robust project foundation
After that lightbulb conversation, Mamdani worked hard to build a robust foundation for this work with colleagues in information technology and research. He got the greenlight to bring in a major commercial vendor for a three-year project to create a mammoth data warehouse that currently integrates nine source data systems with 17,300+ data elements involving hundreds of thousands of inpatient and outpatient visits annually at St. Michael’s. The human mind boggles; the machine learner not so.
The first phase of the infrastructure build was completed in March 2018. Now the drive is on to create data-driven value, projects to generate and apply reliable and useful clinical insights from that enormous pipeline of real-time data.
Ensure clinicians are drivers
What will be acceptable to busy clinicians? That is the central question in an applied healthcare solutions lab like Mamdani’s where the marriage of academic rigor and clinical relevance is sacrosanct. AI-driven data and analysis have to be rock solid: as good or better than human judgment. And how you present that information must be clinically intuitive and – the holy grail – lead to better patient outcomes.
For non-physician health professionals, such as clinical case managers, tools like post-surgical length-of-stay predictors can also help level the hierarchical playing field. Who can argue when they point to reliable analysis to help improve coordination for patients?
“Some physicians are terrified, but in an academic setting most tend to be intrigued by the potential of fields like advanced analytics and data visualization,” says Mamdani, himself a silo buster in his own academic training, earning a Doctor of Pharmacy, Master of Arts in Economics and Master of Public Health.
No ideas without accountability
Mamdani’s team currently has more than a dozen applied analytics projects on the go. Clinicians and administrators pitch ideas for new projects using a proposal template (link) with one important feature: the template requires approval from the relevant program director, medical director and division/department head. Ideas that make it through the filter (a senior-level advisory committee) must have that leadership buy-in to be implemented.
“The potential for artificial intelligence to help our patients live longer, healthier lives is enormous, especially when our partner hospitals work together in achieving this common goal. I can’t think of a better place than Toronto to turn potential into reality,” said Mamdani. The Dec. 3 DASH Summit is invitation-only but for more information on Mamdani’s team of data scientists, contact chart@smh.ca.
News
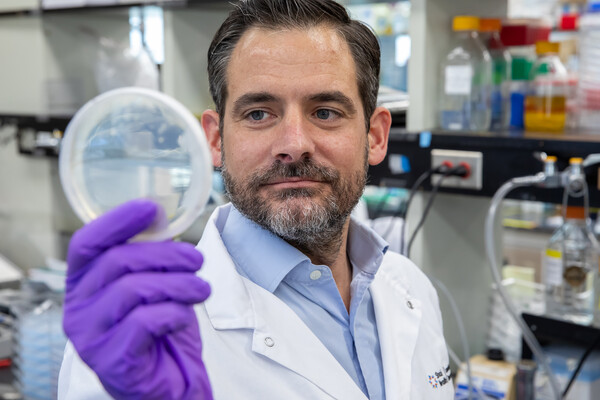

